Now that we have explored some of the descriptive statistics and important basic data values in quantitative research and begun an exploration of inferential statistics, let’s make sure we can sort these concepts out before moving forward.
We have units of analysis about which we collect our data. The data we collect are categorized. Data with categories that can be different from one person to the next or in one set of circumstances to the next are variables. The categories that can be selected for each variable determine the scale of measurement of the data. The scale of measurement determines what measure of central tendency or average you can use, as well as the type of variability measurements that are valid. Those values are relevant both in the display of the descriptive statistics, as those measurements let you know what is normal or typical, and because they are part of many of the formulas in inferential statistical analysis.
Perhaps the two most important inferential statistics measurements are hypothesis tests and correlation strength tests. Most of the time, the formulas for the two tests are one and the same. Sometimes, you need different tests for different purposes.
1) How does the hypothesis test analysis differ from the correlation strength analysis? What do each of these tests tell you about the data you have? Is either of the tests more important than the other? Should you do one type of test first?
2) Some students get bummed out because they find that their p-value is greater than 0.05. What does this mean? Do you believe this would be a valid reason to be unhappy about the data that you have collected? Why or why not?
3) What is the difference between data and information? What sets data and information apart from “knowledge” as we have discussed previously?
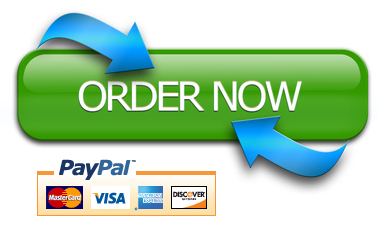